The “ChatGPT Moment”, compared to having been as big as the “Browser Moment” and “iPhone Movement”, was when users all around the world gained access to OpenAI’s ChatGPT model in late November 2022. Within five days, the chatbot had attracted over one million users. Despite the rapid surge in enthusiasm for ChatGPT, the excitement was fleeting, lasting only about 12 months. By 2023, attention had shifted towards exploring AI’s broader capabilities beyond ChatGPT, and the technology industry faced a significant rise in tech layoffs, which were 59% higher compared to 2022.
This shift in focus and industry challenges coincided with a change in public perception. The initial AI fervour began to wane as reality set in. Many are feeling disillusioned, realising that chatbots and language models aren’t the omniscient oracles they once imagined. This disillusionment stems largely from a narrow focus on conversational AI, which, while impressive, has clear limitations. However, this perspective overlooks the broader potential of AI technology. Beyond chat interfaces, these powerful models are driving advancements in many different industries. By shifting our gaze from chatbots to the numerous other applications of AI, this disillusionment shouldn’t detract us from AI’s real potential, as we may better understand how powerful AI models have far-reaching implications across various sectors and scenarios.
Indeed, as we move further into the digital age, the capabilities of AI are growing at a rapid pace and are going far beyond the familiar territory of generative AI chatbots. While the initial hype may have subsided, the true potential of AI is only beginning to be realised. Large Language Models (LLMs) are the leading AI solution in 2024. Outside of image generation, many machine learning algorithms have been replaced by using powerful language models instead of training models on specific data. LLMs are trained on massive amounts of text scraped from the internet and can generate coherent text by exploiting statistical patterns found over such a large set of data.
In 2024, many people think of AI as just a chat bot. Even just focusing on LLMs, it’s possible for AI to do a lot of practical work beyond answering questions in a chat. Achieving business goals typically involves specialised AI apps or custom-built solutions. This can make it difficult to understand what AI can do and what its limitations are. We worked with our AI Engineering team to map out 6 practical business capabilities of AI 2024, including any limits and challenges when using AI in practice.
Practical business applications for AI
In the realm of B2B applications, AI is transforming how organisations operate and deliver value. Businesses are leveraging AI to streamline various processes, enhance customer interactions and gain deeper insights from their data.
1. Customer Service
We've all been there: stuck in a loop with an automated system that just doesn't understand our needs. One of our team members at Affinda recently shared a particularly exasperating experience:
Imagine trying to report a case of fraud, only to be met with a chatbot that stubbornly insists on sending you FAQ links about ‘privacy’ and ‘security’. As if that wasn't frustrating enough, our colleague found themselves trapped in this digital maze with no escape route to a human representative.
“The worst part was that I couldn’t find any way to speak to a human.”
This example highlights a critical flaw in many automated customer service systems: the lack of flexibility and human touch when dealing with complex or urgent issues. While chatbots can be efficient for handling simple queries, they often fall short when confronted with nuanced problems that require empathy and critical thinking.
Another example is the famous DPD chatbot incident occurred in early 2024 when Musician Ashley Beauchamp realised that the courier's chatbot could be manipulated. The chatbot descended into swearing and terrible poetry as he publicly shamed the company on X. DPD responded to the incident saying an error occurred after a system update and the AI element was disabled and being updated.
What makes these experiences so frustrating to customers and how can we make it better? The key challenges of implementing a chatbot in 2024 are:
- Large Language Models (LLMs) continue to have trouble with nuance or multi-step reasoning
- Hallucinations at best cause customer frustration but at worse could cause significant cost to a business, such as Air Canada who were ordered to pay compensation when its chatbot gave a customer false information
- To avoid hallucinations, chatbots often refer customers to existing content that fails to answer their actual questions or address their concerns
- Chatbots are implemented to save on customer service costs and organisations try hard to prevent customers from speaking to a human
It’s important to understand that LLMs need an opportunity to ‘think’ about their answers. The only way these models can think is by generating text. The next thing to understand is that you can give an LLM additional context through Retrieval Augmented Generation, or RAG. Advanced techniques include generating an internal representation of the question (called an embedding) and comparing it to the embeddings of an internal knowledge base. It can also be powerful to ask the model to perform a search just like a human would if they didn’t know the answer.
Here are two real example responses from state-of-the-art LLMs:
In the first example, the LLM is happy to make assumptions and fabricate information, despite its instructions to answer truthfully. The next step is to add the search results into the prompt so that the model can (a) link to the content, or (b) provide a summary to the customer. But be careful! Even with this step it’s still possible for the model to hallucinate and provide a misleading summary of the content. There's also no guarantee that the information found in the search actually answers the customer’s question.
Remember: an LLM needs a chance to ‘think’ by generating extra content you don’t (necessarily) send to the customer.
Implementing Large Language Models (LLMs) in customer service requires a thoughtful approach. Rather than allowing the AI to respond directly to customer inquiries, it's crucial to incorporate multiple steps, including self-reflection and verification where possible. While AI can indeed enhance the customer experience, it demands a deep understanding of the strengths and limitations of modern LLMs.
Assuming these models can comprehend and address customer concerns without proper safeguards is a risky proposition. The key to success lies in setting up your own guardrails and carefully crafting the AI's response process. By doing so, you can leverage AI technology to create a compelling and reliable customer service experience, one that combines the efficiency of automation with the accuracy and care your customers deserve.
2. Image Generation
In recent years, AI has made remarkable progress in generating images from scratch. While the technology is still in its infancy, it holds immense potential for the future of marketing. AI can now create realistic images of products, people, and virtually anything imaginable, reducing the need to spend thousands of dollars on graphic designers or endure weeks of creation for a single campaign image. As of August 2023, people have generated almost 15.5 billion AI-generated images, and each day sees approximately 34 million new AI-generated images.
In saying this, the success of image generation is heavily influenced by the prompt's quality and the specific LLM employed by the AI image generator. Despite the availability of AI image generation, it remains in its early phases with certain limitations. The key challenge is that current AI image generators don’t natively work with layers, and so if you want layering, you need a design tool that allows you to do layering manually.
Additional limitations in 2024 include:
- Inaccuracies and bias due to the data the models are trained on, evident in Gemini’s recent blunder where it produced historical images riddles with inaccuracies.
- Accurately capturing a human: most AI image generators have difficulties capturing the nuances of human features, such as where arms should be and what eyes should look like.
- The ability to add certain features to AI produced images is limited, such as asking it to include a company’s logo. This also raises the concern of deepfakes, with many models implementing policies to deter users from producing images that can be used for cyber attacks, to spread fake news, steal identifies or scam unknowing victims.
- Some LLMS, such as Canva, have character limitations. This means you’re unable to engineer a prompt longer than 280 characters, which is way too short.
- Editing existing prompts: most models have no way to edit existing image outputs based on text, so images produced generally require further editing from a skilled professional.
To understand these limitations further, let’s compare a basic image generation tool, such as Canva’s free online AI image generator, to a more sophisticated one such as Ideogram.

.png)
In the first example, it's evident that Canva’s model struggles with accurately depicting the complexity of a collage and its layers, resulting in limited color usage, misplaced coffee cups, and other inaccuracies. In contrast, Ideogram successfully captures the full extent of the prompt, producing a usable collage image that requires less editing compared to Canva’s option.
The second example highlights Canva’s character limit constraints. Without an optimal prompt, Canva produced an image with two cats (one of which isn’t sleeping) and awkwardly placed a book and tea on the floor, along with other inconsistencies. Since Ideogram has no character limit, it generated a compelling image that accurately reflects the text prompt, including details like raindrops on the window, flowers outside in the garden and family photographs above the mantle.
When comparing AI image generators, Ideogram distinguishes itself through its exceptional ability to accurately interpret and execute user instructions. While Canva's AI functionality has certain constraints that may affect output quality, there's value in utilising both Ideogram and Canva in tandem. Canva's extensive template library, in particular, proves to be a significant asset.
An effective strategy involves merging Canva's Instagram post templates with Ideogram-generated images. This approach yields posts that are both unique and tailored to your specific business requirements. Although Ideogram can potentially create entire posts, if you're seeking greater control over the final product you may prefer to combine AI-generated elements with more traditional design tools like Canva or Photoshop.
To illustrate this concept, we experimented with creating an Instagram post promoting a yoga instructor. This practical example demonstrates how blending AI capabilities with conventional design tools can produce compelling visual content.
.png)
.png)
While AI image generation tools can create images in mere seconds, they do have their limitations as outlined in all three examples above. These tools rely heavily on the quality of the images and tags they were trained on. Their visual output is restricted due to a limited understanding of context, the complexity of the necessary textual descriptions, and challenges in generating intricate, realistic images. Currently, AI-generated images still need to be edited by a skilled professional. Even asking Ideogram to apply text in the Instagram post did not quite work in both examples. However, with the fast pace of advancements in this technology, it won't be long before anyone can create any type of image needed for marketing without requiring any edits.
3. Information Extraction
Information extraction refers to the process where businesses use Optical Character Recognition (OCR) technology in conjunction with AI to read and extract data from various types of documents. Modern OCR systems can now decipher handwriting, understand multiple languages, and even classify extracted data, allowing organisations to use the data as needed through fast document processing. AI document processing automation enables businesses to minimise manual data entry, automate repetitive tasks and lower overall operational costs. But how exactly does it work, and what limitations might it have?
Here’s an example of how Affinda’s AI data extractor can scan and extract all the required data from a purchase order:

There are still major challenges when extracting information from documents and it usually involves some trade-offs:
- Large Language Models are powerful but slow and expensive. You can get more efficient outputs using Small Language Models (SMLs) but they often make more mistakes because they 'understand’ less of the context. Small models usually require ‘fine-tuning’ to become experts at that particular type of data – but this involves collecting annotated data.
- A lot of information about a document is contained in the layout so it’s important to use models which can ‘see’ the document (e.g. to understand which column of a table is a ‘unit price’ vs a ‘total’). As trade-off, images take longer to process and the model may hallucinate more when reading text from the pixels of an image.
It’s typically not enough to send a document to LLMs like Chat-GPT or Claude and hope that they will truthfully extract the information you need. To help meet these challenges, the Affinda team engages clients in a detailed conversation where our AI experts work with organisations to understand which types of models are best suited to solving their unique business needs.
4. Code generation
AI continues to reshape the software development landscape. AI-powered code generation tools have become increasingly sohisticated, offering developers and engineers unprecedented assistance in writing, debugging and optimising code. These systems can now tackle complex programming tasks across various languages and frameworks, significantly accelerating the development process.
However, while AI code generators have made remarkable strides, they are not without limitations. In the table below we explore the current state of AI code generation in 2024, highlighting its capabilities and limitations, drawing from our Engineering team’s firsthand experience in modern software development.
“Right now, a human still needs to understand the business requirements, architect the solution, and review the code written by AI. It’s unsafe to blindly trust code written by AI.”
Capabilities and limitations
Our Engineering team note that AI code generators sometimes produce potentially harmful code, such as commands that delete database entries. In the example below, an engineer demonstrates how GitHub Copilot disregarded explicit text comments warning against dangerous operations, proceeding to generate precisely the risky code it was instructed to avoid.

Automated AI engineer
Several projects, both commercial and open-source, market themselves as ‘automated AI engineers’, promising iterative code development until task completion. However, our practical experience suggests these tools demand significant user input and oversight to be truly effective. Our Engineering team reports occasional use of such systems for routine and straightforward tasks but emphasises that human supervision remains essential for review and validation of the output. The promise of fully autonomous coding solutions has yet to be fully realised, with current implementations still requiring substantial human guidance to ensure quality and correctness.
5. Data analysis
In the realm of business intelligence, AI-driven data analysis is transforming how companies extract value from their information assets. As of 2024, AI demonstrates remarkable capabilities in processing vast amounts of data quickly, identifying patterns and trends that humans might miss. This technology excels in providing predictive analytics, forecasting future trends based on historical data, which enables businesses to make proactive decisions. AI also automates routine analysis tasks, freeing up human analysts for more strategic work and increasing overall productivity. One of its key strengths is offering real-time insights, enabling faster decision-making in rapidly changing business environments.
AI can also integrate data from multiple sources, providing a holistic view of a business’ operations that was previously difficult to achieve. This comprehensive approach to data analysis allows companies to understand hidden correlations and gain deeper insights into their operations, customers, and market trends.
This data analysis can be separated into two different categories: qualitative vs quantitative. Here’s an example at how AI can help with data analysis in both categories.
Qualitative:
In the context of B2B services, one compelling example of AI application is in the field of legal discovery. Legal discovery can be extremely labour-intensive for legal professionals, especially when it comes to complex cases. Searching through hundreds or thousands of documents that may or may not be useful is not only time-consuming but also requires meticulous attention to detail to avoid overlooking crucial information. Leveraging AI for legal discovery can help perform discovery functions including:
- Automate data collection, processing, and review
- Craft summaries of legal documents or data sets
- Review electronically stored information (ESI): devices, cloud, systems, social, public, etc.
- Identify unexpected patterns and connections within data
- Present clustered data in interactive visual charts
- Assess and label photos
For example, an AI tool designed for legal discovery might flag a series of emails discussing a particular financial transaction relevant to a fraud investigation. Instead of merely highlighting the occurrence of keywords like "transaction" or "fraud," the AI could provide extracted sentences or paragraphs that show the context in which these terms were used, ensuring that the legal team can quickly understand why these documents are significant.
Additionally, these AI systems can be trained and iteratively improved based on feedback from legal experts. As the AI processes more documents and receives guidance from a human on what constitutes relevant evidence, it becomes increasingly adept at identifying key issues and reducing the likelihood of missing important information.
Although AI is readily available to help in this space, there are still key challenges that legal professionals face when using AI for legal discovery, or for any legal processes:
- Hallucinations – there is a need to have proper grounding of responses when using AI for law or legal processes to avoid hallucinations. Allen’s 2024 Australian Law Benchmark for Generative AI found that AI models should not be used for Australian law legal advice without expert human supervision, as there are real risks to using them if you don’t already know the answer. While there are retrieval augmented generation (RAG) methods that claim to ‘eliminate’ or ‘avoid’ hallucinations, Arxiv’s 2024 report assessing the reliability of leading AI legal research tools found that while hallucinations are reduced relative to general-purpose chatbots (GPT-4), the AI research tools made by LexisNexis (Lexis+ AI) and Thomson Reuters (Westlaw AI-Assisted Research and Ask Practical Law AI) each hallucinate between 17% and 33% of the time.
- Bias – LLMs are not pure objective engines of reason as they have been trained by humans. This means that the people who train them can be biased, and thus AI models can also be biased.
- Input risks – the greatest risk of using a LLM in the legal industry today is a potential breach of confidentiality. If precautions aren't’ taken, AI models could wreak havoc on attorney-client privilege and client data security obligations.
- Ethics – Thomson Reuters cover the primary ethical pitfalls of using generative AI and LLMs in law, such as misunderstanding the technology and failure to protect confidentiality.
Quantitative:
With the risk of hallucinations, you wouldn’t simply paste raw data into an LLM chat box and hope it provides you with a decent analysis. Instead, you want your LLM to write code which analyses the data for you. Writing code for data analysis is a big strength of modern LLMs. Combined with the ability to understand natural language queries, you have a tool which can turn questions into code.
Familiar problems persist, however – you need access to the data and the ability to run and write code. In large organisations this might mean access to business intelligence tools which have restrictions for Personal Identifiable Information (PII) or commercially sensitive business data. For smaller organisations, it means getting data out of common tools like CRMs and into useful data analysis platforms.
For even medium-sized datasets there may be hundreds of tables and thousands of columns which means engineering prompts to tell the models exactly what information is available. This means that doing data analysis with AI isn’t going to be accessible to most people.
“For now, AI driven data analysis is just another tool for those already skilled in data analysis.”
Let’s consider a seemingly straightforward question: “which existing customers have the most potential for increased sales?”
An ideal AI model should first clarify its interpretation of the question. For example, it might propose: “Let's identify customers who have made significant purchases but across a limited range of product categories.”
However, this interpretation alone isn't sufficient. Even if we accept this approach, how can we ensure the data used accurately addresses the question? Consider this AI interface that outlines its analytical process, the steps used to answer the above question:
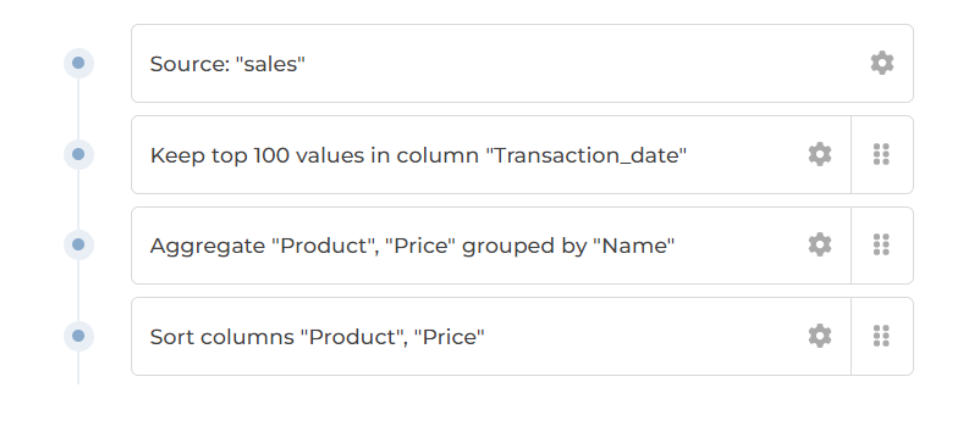
This raises important questions. For example, why has it chosen to limit the analysis to only the 100 most recent transactions? Without access to the underlying logic, it’s impossible to identify potential hidden assumptions or mistakes that the model may have made. While tools like Microsoft’s Power BI Copilot are moving in the right direction, user feedback suggests it still requires very specific instructions to provide any valuable analysis. This often demands prior familiarity with Power BI or similar tools.
6. Content Marketing
The content marketing landscape has undergone a seismic shift with the advent of AI. Gone are the days when marketers spend countless hours brainstorming ideas, crafting articles, and searching for the perfect images. AI has ushered in a new era of content creation that is faster, more personalised and increasingly sophisticated.
The biggest challenge for content marketing, however, is that modern AI produces reasonable sounding but often shallow content. Without extra work LLMs can’t perform research on their own so they are best at producing content that features heavily in its training data. Consider these two examples asking the same model about writing a social media post about (1) travelling in Bali, (2) content marketing.
It’s not hard for an AI to write something interesting about travelling to Bali because it’s a straightforward topic that has a lot of content written about it that is readily available online. The post about content marketing, however, says to focus on high-value customised content, but the post it’s written is low-value and generic.
This showcases AI-powered content’s challenges and limitations in 2024:
- Authenticity and originality: While AI-generated content has improved dramatically, it can still lack the authentic voice and original insights that come from personal experience.
- Legal and ethical concerns: The use of AI-generated images raises copyright and ethical questions, particularly when creating images of real places or people.
- Over-reliance risk: There's a danger of content becoming too homogenised if many marketers rely on similar AI tools without adding unique human perspectives.
- Emotional nuance: AI still struggles with conveying complex emotions or cultural nuances that human creators intuitively understand.
- Fact-checking necessity: AI can occasionally generate plausible-sounding but inaccurate information, requiring human verification.
- Research: Most AI models are incapable of performing in-depth research, resulting in content that is often shallow and generalised, as it relies solely on the data the model was trained on. However, using a model like Perplexity can enhance content marketing efforts. Perplexity is an AI chatbot-powered research and conversational search engine that generates answers by sourcing information from the web.
Imagine a world where a marketing team can produce a month's worth of content in a single day, complete with tailored articles, eye-catching visuals, and data-driven insights. While AI tools in 2024 may assist in content marketing, their capabilities are still limited. However, in the not-so-distant future, AI could become the ultimate creative collaborator, enhancing human creativity with machine efficiency and opening up possibilities that were once thought impossible.
Looking forward: AI in the future
As we peer into the future of AI, one emerging trend stands out: agent-based AI. We're seeing early signs of this with platforms like Perplexity, which hint at a more interactive and autonomous form of artificial intelligence. These AI agents are poised to transform how we interact with technology and information. In the near future, we can expect to see AI agents interacting not just with humans, but with other AI agents as well. This opens up exciting possibilities, particularly in areas like product research. Imagine multiple AI agents collaborating to gather, analyse, and synthesise information from various sources, providing us with comprehensive insights in record time. The core driver behind these advancements is the continuous improvement in natural language processing and understanding. As AI becomes more adept at interpreting and generating human-like text, its ability to act as an autonomous agent will only grow stronger.
It's worth noting that while there may have been some initial disillusionment as AI technologies were introduced to the mainstream, the field continues to evolve at a rapid pace. From automating customer service to assisting with powerful content marketing, AI is proving its worth across numerous sectors, offering a multitude of practical and innovative solutions. As we move forward, it's clear that AI will play an increasingly significant role in the business landscape. While challenges remain, the potential benefits are immense. By staying informed and adaptable, we can use the power of AI to create more efficient, innovative, and user-friendly experiences in the years to come.